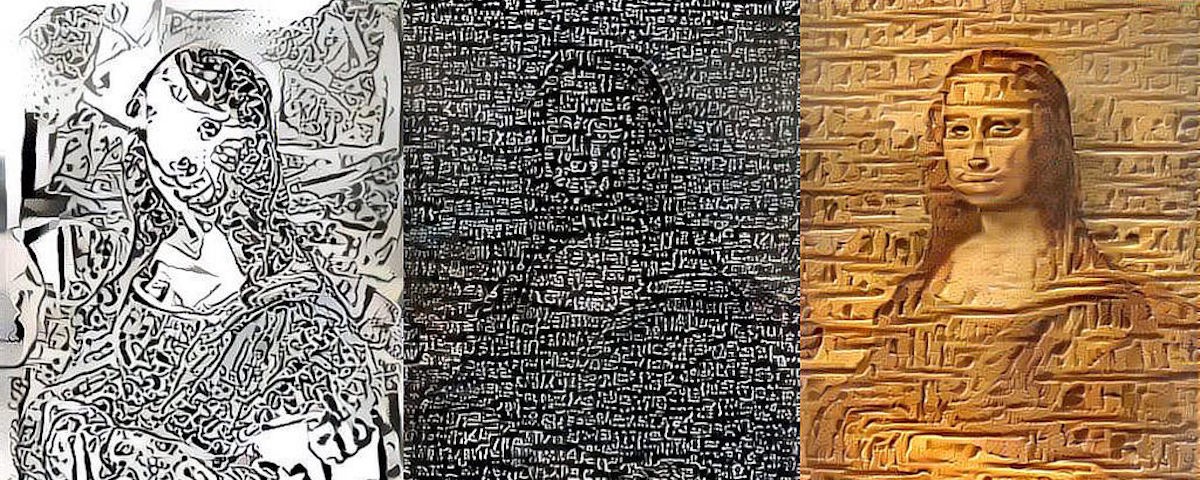
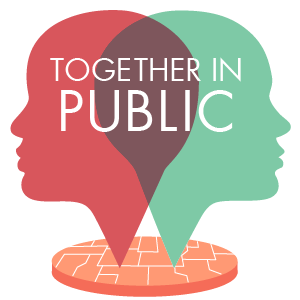
Though characterizations of a “Twitter revolution” are somewhat trite, it’s widely acknowledged that social media played a big role in the 2011 Arab Spring. Online commentary from citizens across the Arab world not only narrated, but shaped and orchestrated events in the physical world as they unfolded.
The extent to which social media helped bring regime change in the region received extensive (some have argued exaggerated) coverage in Western media at the time, as did discussion of the importance of the Arab blogosphere in forming the discourse of a new generation of activists. For politically engaged internet users–myself included–it was impossible to avoid regular online and offline discussions about the new popular consciousness being forged across the digital networks of the Middle East.
What I didn’t encounter was any suggestion that English-speaking internet users could venture into this world ourselves, even as observers. I don’t remember anyone in my circle sharing links to Arabic-language blogs that could be translated into other languages, although I do recall seeing interviews with English-speaking bloggers broadcast on television news. This reflects the fact that the promise of the internet–the world as a global village, with a free exchange of information across borders and cultures–is in practice very much limited by the bounds of language.
Think for a moment about what you even consider the internet to be, and it’s more than likely you’re imagining a narrow group of websites and services in the language(s) you speak. The portion of the internet an average Russian speaker visits, for example, is almost completely separate from the internet seen by a Brazilian; the Chinese internet is cut off from the Anglophone world not just by the Great Firewall, but by the sheer impenetrability of the characters for anyone accustomed to Latin script.
With the Arab Spring, my impression was that it was only the bloggers who spoke proficient English (or perhaps French) who piqued the interest of European media producers and consumers. Dalia Othman, a researcher on the Middle East and co-author of a paper on the structure of the networked public sphere in the Arab region, confirms that I wasn’t wrong in this estimation, telling me that English-speaking bloggers acted as liaisons between Arabic and Western media. She says that in many cases online content from the Arab world was (and still is) created in English to have broader appeal.
This feature from The Guardian charts some of the quantifiable evidence of this divergence: Twitter users overwhelmingly follow, retweet, and reply to users who speak their native language; Google maps will suggest different locations depending on whether the word “restaurant” is searched in Hebrew or Arabic; and even on Wikipedia, with its grand vision of creating a shared repository of global knowledge, 74 percent of concepts listed have entries in only one language.
While automatic translation is sometimes used in the context of informal conversations such as Facebook comments, Othman also notes that mistranslations can leave meaning mangled: “In most cases it’s obvious that they’ve done some form of translation because they’re not getting the full meaning of the post. And that’s because, I have to be honest, so far [automatic] English to Arabic translation is a disaster–and this is saying it as someone who’s tested it out.”
Part of the difficulty stems from the heterogenous nature of Arabic as a language–it has many different regional variations with far greater differences than English dialects. When Google offers Arabic translation, it’s to or from Modern Standard Arabic–a formalized version of the language–which leads to errors if a post is written in, say, the Egyptian vernacular. (Journalism translation provider Meedan raised this point back in 2012, which led the company to build products around the use of crowdsourcing to quickly translate Arabic social media content into English.)
In many respects, increased linguistic fragmentation is just a sign of the growing diversity of the net. In the mid-1990s, English made up an estimated 80 percent of all online content; although English is still the most represented language online today, the last two decades have seen a blossoming of Chinese and Spanish as well, with Arabic and Portuguese now fourth and fifth most common, respectively.
Still, it’s hard not to feel that a world in which we are all online, but only talking within our distinct language and culture groups, is somehow falling short of the utopian vision of the internet exemplified by John Perry Barlow’s famous decree: “We will create a civilization of the Mind in Cyberspace. May it be more humane and fair than the world your governments have made before.”
Creating any kind of singular “civilization of the Mind” requires us to be able to spread ideas as seamlessly as possible among individuals in all corners of the globe. Sending information across huge distances means nothing, though, if it can’t be understood.
We know that translating text online is now as easy as clicking a button–but will automated services ever really be enough to break down the boundaries between us?
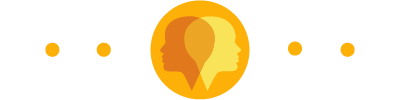
Take this headline and lede from a news story, translated from Turkish into English:
Top Searches on Google in Arab Countries
The search engine Google has searched for the most jobs and business building project in Saudi Arabia this year, high school exam results in Egypt, and the words of the European Football Championship in the United Arab Emirates.
It seems simple enough: a story about search trends in the Arab world, which I took directly from a Turkish news site and ran through Google Translate. It’s good enough for an English speaker to read and understand, even with its few errors. Besides the missing “s” in “projects” and the phrase about users looking for the “words of the European Football Championship” (presumably a better translation would have been “European Football Championship were the most searched words in the United Arab Emirates”), the sentence is grammatically sound. But to a native speaker it’s immediately obvious that something is awry, and its shortcomings are great enough that there is little pleasure to be derived from reading it.
Beyond the challenges for machine translation, there are even bigger problems of linguistic division on the internet. For example, searching for “Turkish news site” only showed me English-language sites operating in Turkey. Much as Google may trumpet the efficiency of Google Translate, its search function still has a near total bias toward returning search results only in the language of the search terms–perhaps a tacit admission that translated results are not yet at a high enough grammatical standard to be acceptable to most users. But before we think about the errors that a machine makes with language, let’s take a moment to ponder how remarkable it is that humans, for the most part, don’t do the same.
On average, human infants will start to produce their first recognizable words at around 12 months old. As parents well know, by as early as three years old many children can hold an imperfect–but frequently hilarious or infuriating–conversation with an adult, and by five, they are using correct grammar and vocabulary in the vast majority of their speech.
As an adult, using a language as a native speaker means effortlessly following an array of rules that dictate what is and is not allowed, even when we’re not aware that we follow them. We can instantly recognize sentences we’ve never seen before as either grammatical or ungrammatical. And then, beyond the grammatical acceptability of a sentence, we know if it makes sense–a distinction that linguists have made their careers on.
With shades of subtlety that are mysterious even to those who grew up speaking a language, how do we go about teaching it to a machine? The answer involves a lot of complex math–and a conception of language that also seems extremely foreign to our experience of speaking or reading it.
“Natural language processing is about modeling human language using computational models,” says Jackie Chi Kit Cheung, assistant professor at McGill University’s School of Computer Science and a specialist in the subject. “In natural language processing we look at what sorts of formal representations, or mathematical constructions, can be used to describe natural language, and how to come up with them. So, for example, finding algorithms for parsing a sentence into verbs, nouns, and other syntactic relations; and [doing] this efficiently and accurately.”
Today, Cheung and most other researchers in the field focus on what’s called a statistical approach to machine translation: building a model based on the probability of words occurring in a certain sequence, given patterns that have been learned from analysis of a large corpus. But the statistical approach hasn’t always been the norm. In fact, it marks a distinct change in methodology since the early days, in which machine translation was much more influenced by conventional linguistics.
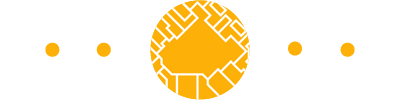
The first ever public demonstration of machine translation was carried out in 1954 at the headquarters of IBM in New York. The New York Times covered the event in a front-page story, writing: “This may be the cumulation of centuries of search by scholars for “‘a mechanical translator.’ So far the system has a vocabulary of only 250 words. But there are no foreseeable limits to the number of words that the device can store or the number of languages it can be directed to translate.”
Besides the programming of the vocabulary, this first IBM system took a rule-based approach to translation, a method which would dominate for the next three decades. The idea was that expert linguists could help to codify the rules of language in such a way as to be programmed into a machine; the machine would then attempt to translate words and sentences, and for each exception or error found a new rule could be added to correct it. (It can be thought of as a similar process to studying a foreign language as an adult, where we attempt to memorize rules and vocabulary in a systematic way and learn from having our errors pointed out.)
After the initial buzz, progress along these lines was slow but steady through the 50s and 60s, until a damaging report published in 1966 put a damper on research and funding by concluding that machine translation had proved more costly and less accurate than employing human translators, with little prospect for improvement in the near future. Certain key research groups did continue their work, but on the whole computational linguistics as a discipline fell out of favor until the 80s when the nascent microcomputer industry rekindled interest in the automatic translation of digital text.
In the early 90s, research breakthroughs and increases in processing power allowed for the kind of data analysis that would make the first statistical translation system possible, and, coupled with the internet explosion of the latter half of the decade, the late 90s saw the first forays into machine translation online. Probably the most iconic of these was AltaVista’s Babelfish, a service which provided two-way text translation between English and French, German, Spanish, Portuguese, and Italian, along with the ability to enter a web URL and have the whole page translated. Anyone who used this service at the time will recall how magical this seemed.
Google, a comparatively late entrant into the field in 2006, has now come to dominate the market for free online translation. According to Google’s own figures, the Translate service processes more than 100 billion words per day, with support for more than 100 different languages in text form, 24 of which can be translated through speech recognition. The most common are between English and Spanish, Arabic, Russian, Portuguese, and Indonesian, with Brazilians using the service more than any other country.
Stories about how Google Translate is bringing unlikely people together have an undeniably feel-good quality to them, connecting into a narrative about the bridging power of technology that we’d all like to believe. But do those 100 billion daily words really get us closer to understanding each other when the stakes are high?
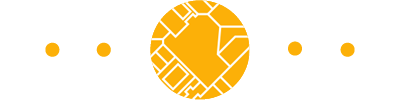
You probably don’t know the name, but SYSTRAN has been a force in the field of machine translation for almost half a century. Founded in 1968, the company started with close ties to the U.S. Air Force, translating Russian documents into English during the Cold War. It was actually SYSTRAN’s software that powered both babelfish.altavista.com and the first version of Google Translate–and the company is still involved in cutting-edge research today. So when SYSTRAN’s CEO and chief scientist Jean Senellart talks about a breakthrough, it’s worth listening.
“I’ve been working for SYSTRAN for 19 years, and I think we’ve never seen something as big as what’s coming in neural machine translation,” Senellart says in the first few minutes of our call. “The breakthrough is so big that in one year, I’d be surprised if there is any alternative to [using] it.”
Neural machine translation (NMT) is the application of neural networks, a subset of machine learning, to the translation process. A more lengthy explanation can be found on the SYSTRAN blog, but briefly, a neural network can analyze a text in such a way as to construct a “thought vector“–an abstract representation of the different semantic elements of the source text–which can then be rendered in multiple different output languages, instead of needing to work with language pairs. Not only that, but the accuracy and fluency of the translation is striking. Having looked at some examples of NMT between English and French, it’s hard not to feel that the system understands how to construct a natural-sounding sentence.
Senellart is not the only one excited about neural machine translation. In a blog post from November 2016, Google Translate product lead Barak Turovsky described the switch as “improving more in a single leap than we’ve seen in the last 10 years combined.” (Google has slowly begun to roll out NMT for different language pairs in the time since then.) In the specific case of English-Arabic translation, research on NMT is scarce at present. One research team concluded that it produced comparable results to phrase-based translation in some circumstances and significantly outperformed it in others; as more research is done, there’s no reason to think that gains for Arabic will not be as great as for other languages.
So how will this impact our internet experience? Senellart describes a vision is for an internet where displaying the subtleties of translation becomes a standard part of the user experience. “I think that we’re going towards true multilingualism,” he says. “Today when you want to get information from a Chinese website, you translate the site, and it’s no longer in Chinese–it’s in French, English, whatever you’re looking for. Tomorrow, I think we’ll be able to look at a webpage in Chinese and still see the Chinese “¦ but see the ambiguity that is in the language, in your own language. The machine will help us to decipher it.”
Of course, as with the example of translation (or lack of) during the Arab Spring, we know that the ability to do something does not on its own equate to the will to do it. We’re within striking distance of a level of automatic translation that starts to really convey some of the enormous subtleties that human language is capable of, but at the same time, it would be naïve to think that fractures between online language communities could be solved by purely technical means.
In the wake of the U.S. election, it became clear that speakers of the same language in the same country can inhabit very separate online spheres. Seen in that light, the vision of a world where a person reads a French news site over breakfast, Facebooks with Russian friends at lunch, and comments on a Japanese forum in the evening still seems a long way off. In fact, our path toward it might depend more on decisions made by the new gatekeepers of online content–the search engines and social media platforms–than it does on the strength of translation. Google, Facebook et. al. will almost certainly experiment with serving translated content in the near future, and reactions to it will be a bellwether for what’s to come.
Machine translation, however good, is not going to be a silver bullet for uniting disparate communities online, but it’s still an amazing addition to our ability to communicate. Perhaps the true civilization of the mind, rather than being a uniform mass of consciousness, is instead a place where we speak different languages, yet are still understood.

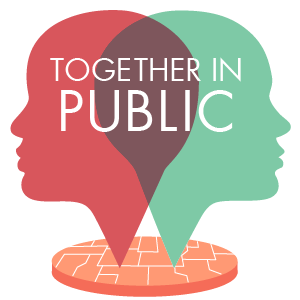
How We Get To Next was a magazine that explored the future of science, technology, and culture from 2014 to 2019. This article is part of our Together in Public section, on the way new technologies are changing how we interact with each other in physical and digital spaces. Click the logo to read more.